What is Big Data?
Nowadays, it is no longer a surprise that almost every Internet service attempts to determine the desires of users in a very short time and offers products, movies, music, and other types of content. This would not be possible without analyzing colossal data. In recent years, there has been a real boom in Big Data, and today many processes rely on it.
In 2008, Clifford Lynch proposed the term Big Data in his article in the journal Nature. He spoke about the global growth of information volumes in the world and noted that new and more developed tools will help master them.
Since then, the term has defined this concept as structured or unstructured large volume data sets with a set of methods of storing and processing information for automation, optimization of business processes, and adoption of the most effective decisions. Forrester puts it succinctly, “Big data combines techniques and technologies that extract meaning from data at the extreme limits of practicality.”
The world’s largest data operators, such as Google, Microsoft, IBM, Oracle, Amazon, were first to actively collect user data, analyze it, and use it to improve their services. Thus, big data analysis has opened up the opportunity for companies to study the preferences of their customers and use this information when working on various development strategies.
The information volume of Big Data is constantly increasing. In 2020, it was 44 zettabytes. We are creating data at such a high rate that we have had to invent new words like zettabyte (ZB) to measure it. One ZB is a trillion gigabytes. For example, that is almost 8 billion smartphones with 128 GB memory. If you put all these smartphones one after another, you could circle the Earth 45 times. By 2025, IDC estimates that the total volume of business data will reach 175 zettabytes.
The sources of Big Data involve the Internet of Things (IoT) and connected devices; blogs, social networks, websites, and media; company data: transactions, orders of goods and services, taxi and car sharing trips, customer profiles; various professional databases, including medical, geolocation, meteorological, satellite, cellular data, and others.
According to the Cisco Annual Internet Report research program, the three Vs – volume, velocity and variety – respectively denoting the physical volume, the speed of obtaining new data, and its rapid processing, can explain the principle of Big Data. Subsequently, the principle of three Vs expanded to eight Vs, including veracity, viability, value, variability and visualization.
There are many different techniques for analyzing data sets. McKinsey characterizes data processing methods as follows: crowdsourcing (classification), data integration, machine learning, and network analysis using genetic algorithms, pattern recognition, predictive analytics, simulation modeling, spatial analysis, statistical analysis, and visualization of analytical data.
Where is Big Data Used?
The potential of Big Data analytics is so great that today it can be used in many industries, such as manufacturing, medicine, retail, telecommunications, etc.
More than 55% of companies in the US now work with big data. Over the past five years, the use of Big Data in business has tripled.
For example, a manufacturer can predict demand for its products based on historical sales, seasonality of demand, market conditions, changes in the cost of consumables, and so on.
The main value of using big data in production is cost reduction and optimization. The implementation of Internet of Things-based solutions allows companies to monitor the condition of equipment, prevent possible failures, and simulate production processes. One of the highest indicators of effective use relates to the energy sector. Big Data analytics increases the accuracy of generator power distribution by up to 99%.
The introduction of mobile technologies and the spread of Machine-to-Machine (M2M) devices has accelerated the use of Big Data in healthcare. Analysis of information arrays makes it possible to study the effectiveness of treatment and prevention of diseases, allows to develop new methods for improving population health, and predict the demand for pharmaceuticals. Big data can be used to track the growth of epidemics. Using big data, a group of researchers and scientists from Boston was able to detect the spread of fever in Guinea nine days before the Ebola virus outbreak was officially declared.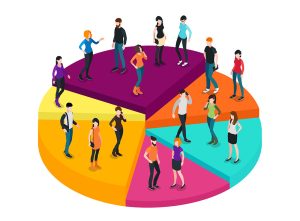
In retail, big data helps companies better understand their customers, optimize logistics and forecast sales. Big Data allows you to segment the audience by more than five thousand parameters, such as the most popular customer locations, customer gender and age, target price segment, interests, and so on. The implementation of big data analysis tools by the world’s largest wholesale and retail chain Walmart allowed the increase in online sales revenue from 10% to 15%. Amazon’s new recommendation system, which is based on Big Data, began to generate a third of the company’s revenue.
Similar to retail, in telecom big data makes it possible to collect more detailed information about consumers and use it to improve service offerings, develop new pricing and services, forecast sales. One of the most useful applications of big data in telecommunications is fraud prevention. Thanks to machine learning technology, operators can track spam calls and protect subscribers from intrusive advertising. Telecom operators are one of the largest owners of big data about transactions, geolocation, search queries, and profiles in the Internet. This allows them to offer Big Data analysis and processing services to companies in other areas.
Risks
Big data has brought not only new opportunities to the public but also new risks.
Technological and organizational problems. Big data is heterogeneous, making it difficult to interpret. The more parameters required for prediction, the more errors are accumulated during analysis.
Thus, according to a study by New Vantage Partners, it was noted that almost 91% of surveyed companies indicate that the biggest problems are difficulties of managing large volumes of information, which are aggravated by organizational problems, lack of personnel, and relevant experience of workers.
Security risks. Storing and processing large amounts of information are associated with increased vulnerability to hacking, which is why they have become an attractive target for cyber-attacks. A striking example is the scandal with Facebook when data leaks of tens of millions of users occurred. User anonymity has become very problematic, which gives rise to several ethical problems associated with privacy. 72% of US adults believe companies have too much control over their data (YouGov).
Social and cultural problems. In many ways, the advantages and capabilities of Big Data depend on data quality, i.e. on reliability and timeliness of information. Experts write that it is not always accurate and sometimes is biased, or its selection is made according to arbitrary criteria.
Current Trends
Big Data has become increasingly popular strategic resource for effective management. As a result, the challenge of quality data management and analysis drives some of the current trends that we see.
- Artificial intelligence has penetrated in many aspects of life. The use of AI technologies, the latest and most advanced, is rapidly becoming mainstream in working with corporate data.
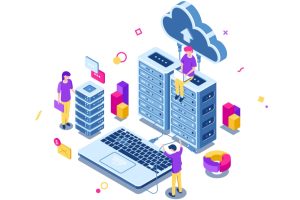
Let’s take the method of Transfer Learning (TL) as an example. It allows you to use the knowledge gained from training models on one problem to solve other problems. Compared to traditional Machine Learning (ML), transfer learning saves a lot of time.
For example, you need different models to detect trucks and buses in images. With a conventional ML approach, you need two datasets to train two different neural networks. The creation of the first model by using transfer learning identifies all auto vehicles, and on its basis, models are made to recognize trucks, buses, limousines, convertibles, tractors, and so on. As a result, we have higher productivity and quality with transfer learning.
- Improving the quality of natural language processing is another trend. Big data, artificial intelligence, the Internet of Things, and machine learning are expanding the boundaries of human and technological interaction. This gives these technologies a human face through Natural Language Processing (NLP).
NLP allows even the most ordinary users to interact with intelligent systems. They do not need to resort to complicated coding. Now, you can query complex data sets using ordinary words and phrases through voice or keyboard input and get the same easy-to-understand business intelligence results.
Further implementation of NLP tools will significantly increase the level of distribution of data mining technologies. For example, it can provide businesses with access to sentiment analysis. This will allow them to learn how their customers feel about their brands on a much deeper level. There are many ways that information can be tied to specific demographics, income levels, education levels, and the like.
- Emphasis on data visualization or dataviz, short for Data Visualization. The human brain can process visual effects 60 thousand times faster than text and numbers. In addition, it can recognize trends, identify potential problems, and predict future developments using visual representations of data such as graphs and charts. The purpose of graphical display is to improve usability and make data easier to understand.
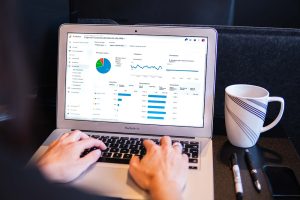
The problem lies at the intersection: on the one hand, you need to have a good understanding of the nature and meaning of your data. On the other hand, you need to have strong UX competencies to visually present this data.
- Data-as-a-service (DaaS) is becoming increasingly ingrained in our lives. The popularity of DaaS as a management concept has grown due to the development of the market of specialized cloud providers that sell analytics results on a subscription basis. This ensures that users get the data they need for their business without extra infrastructure costs or additional staffing.
Essentially, this is an approach to working with data streams, the goal of which is to provide end users with the result of processing data streams in an understandable and easy-to-use form, in a short time and in a manner protected from external interference. The data-as-a-service model works in two ways: 1) volume-based, when payment is made depending on the amount of data, or when the payment is taken for each API call of the consumer to the platform of the data provider; 2) type-based, which is pre-structured by providers, such as geographical, financial, historical data, etc.
It is worth noting that DaaS is used both for the company’s internal needs and to fulfill the tasks set by customers. Moreover, in both cases, DaaS structures the work process, allows you to increase income, and speeds up getting results. Research firm Gartner projects that end-user spending in DaaS will grow 26.6% by the end of 2023.
- Real-time analytics is the need of the hour. Total digitalization has made it possible to receive and process data almost instantly. Managers now want to enable enterprise systems to respond to business events with near-zero latency. For instance, they want not just to learn about the sale that has occurred in a chain store, but also to analyze all sales on the fly and make decisions. For example, cancel a discount if the product is already selling well. Or urgently reduce the price if there is a risk of not selling before the expiration date. It is possible that many industries will work at the same pace as traders on the stock exchange soon.
There are two main reasons for speeding up data analysis. First, many businesses have gone online. People want to know everything and at once. Second, this is the beginning of the widespread penetration of IoT solutions into various industries.
- The exponential growth of information will lead to new solutions in data storage technologies. Many companies are concerned that the volume of data is growing faster than they can store and analyze. Currently, the average share of IT infrastructure spending is about 3-4% of the total budget of US companies, while storage systems can account for up to 30% of total IT spending. This means that approximately 1% of the total budget is spent on data storage. Moreover, if the volume increases 100 times, will it be 100%? How can a business survive then?
Will storage system manufacturers have time to give a worthy response to the impending information explosion? There is no firm answer. For now, suppliers of traditional HDD/SSD dominate the market and do not intend to lose ground. Of course, in the fierce competition, they improve the characteristics of their devices and reduce prices.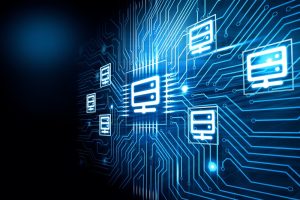
However, due to existing challenges, new recording technologies are becoming more common, such as Shingled Magnetic Recording (SMR), Perpendicular Magnetic Recording (PMR), and Heat-assisted magnetic recording (HAMR). Experts believe that by 2025, the capacity of hard drives will grow to 100 TB thanks to innovations, and the next-generation data storage market will accelerate.
Thus, the big data industry will undoubtedly continue its rapid growth as long as people continue to generate data. New developments in data and analytics will give companies more opportunities to better serve their customers and increase revenue.